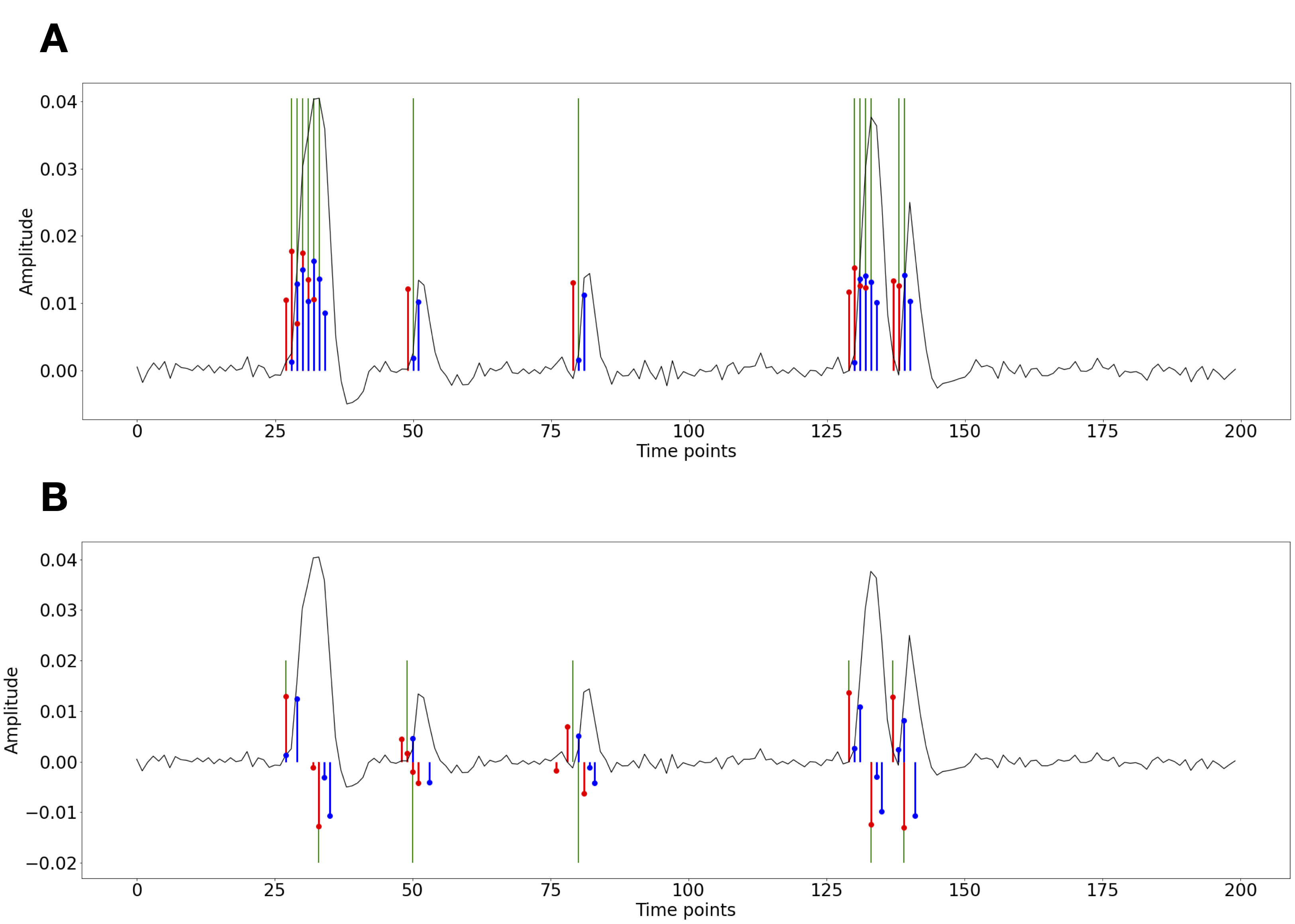
Figure 1:Activity-inducing (A) and innovation (B) signals estimated with PFM (red) and TA (blue) using their built-in HRF as opposed to using the same. The black line depicts the simulated signal, while the green lines indicate the onsets of the simulated neuronal events. X axis shows time in TRs.
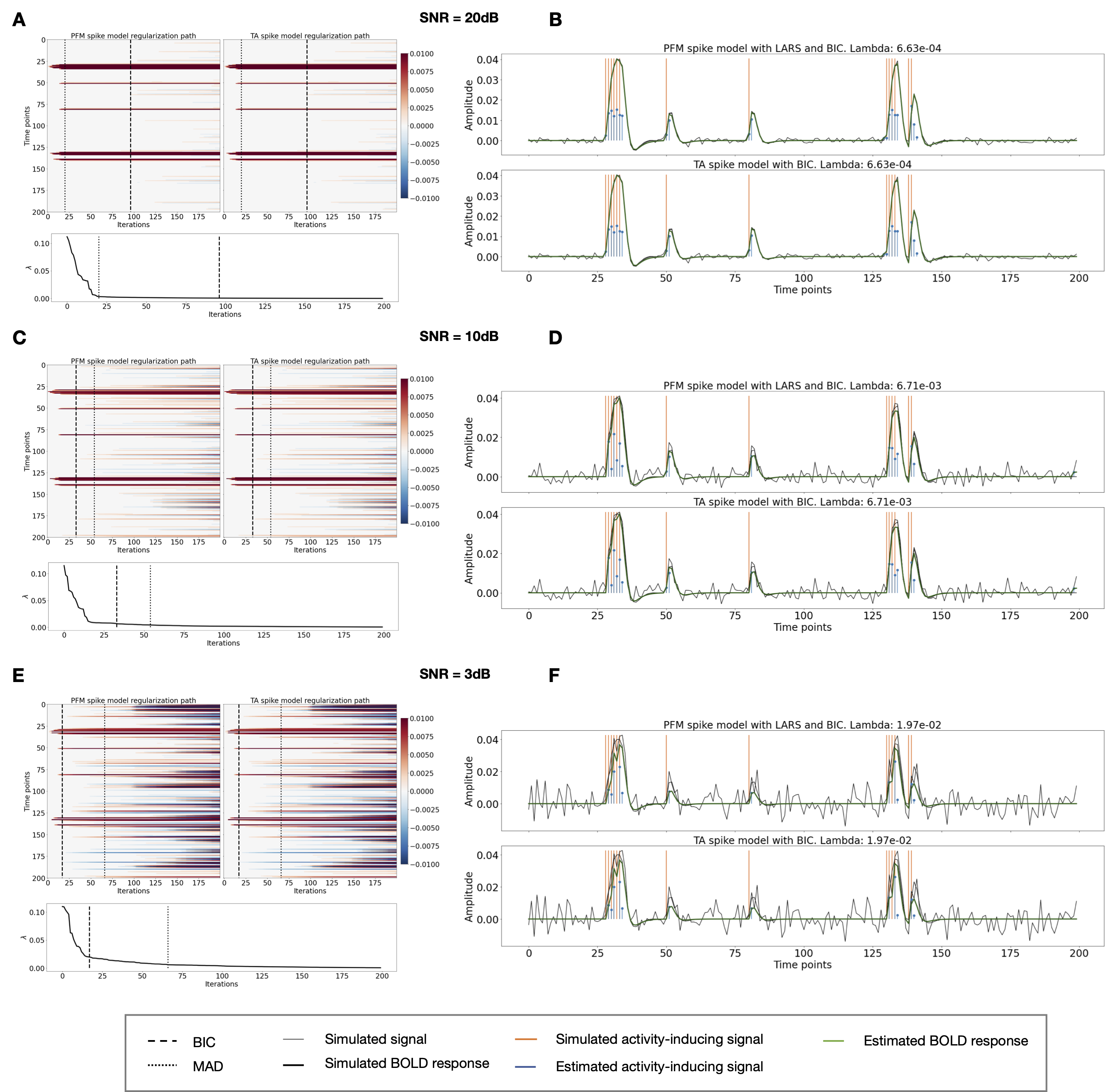
Figure 2:Spike model simulations. (Left) Heatmap of the regularization paths of the activity-inducing signal estimated with PFM and TA as a function of λ (increasing number of iterations in x-axis), whereas each row in the y-axis shows one time-point. Vertical lines denote iterations corresponding to the Akaike and Bayesian Information Criteria (AIC and BIC) optima. (Right) Estimated activity-inducing (blue) and activity-related (green) signals when set based on BIC. All estimates are identical, regardless of SNR.
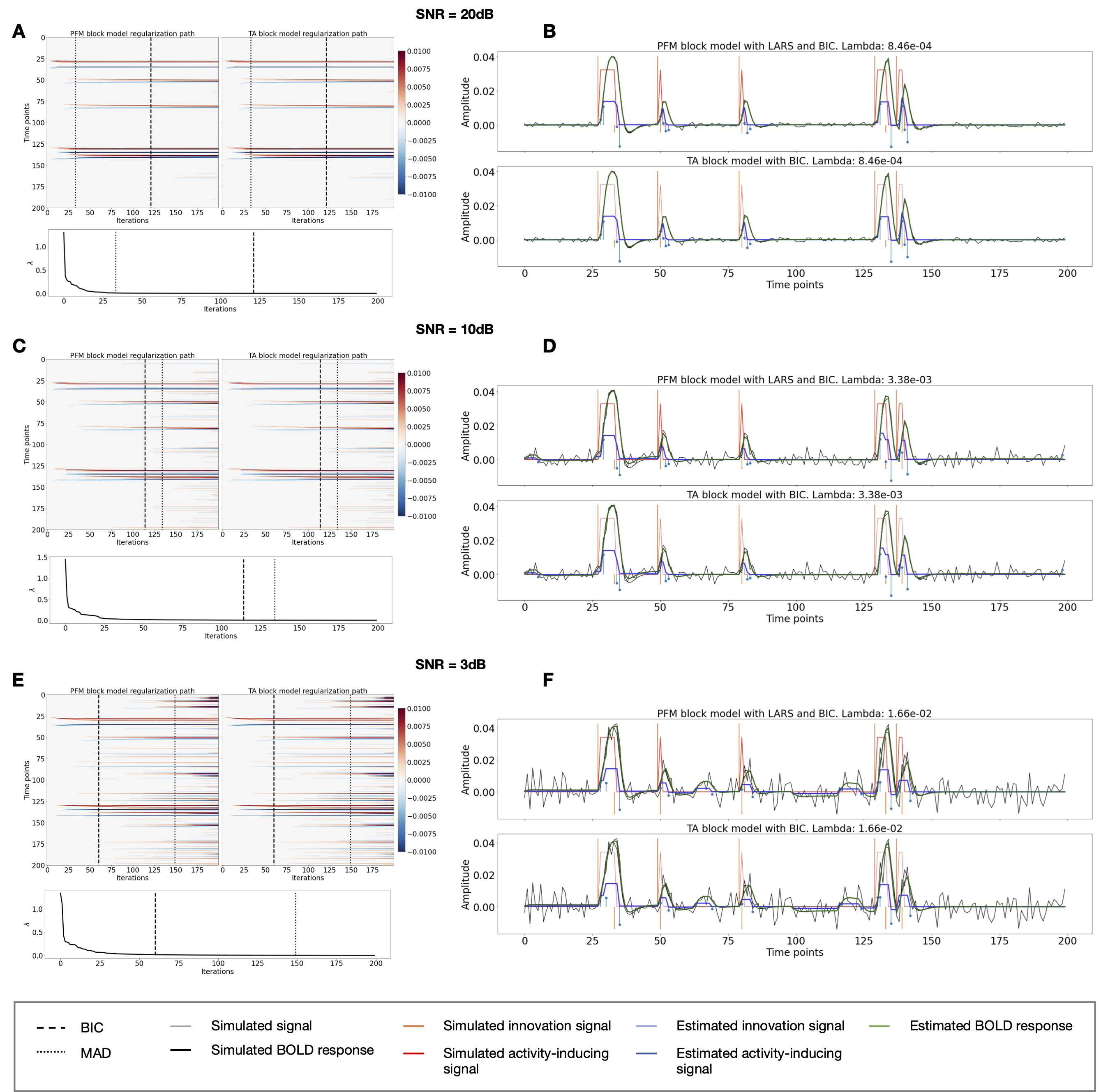
Figure 3:Block model simulations. (Left) Heatmap of the regularization paths of the innovation signal estimated with PFM and TA as a function of λ (increasing number of iterations in x-axis), whereas each row in the y-axis illustrates one time-point. Vertical lines denote iterations corresponding to the Akaike and Bayesian Information Criteria (AIC and BIC) optima. (Right) Estimated innovation (blue) and activity-related (green) signals when is set based on BIC. All the estimates are identical when compared between the PFM and TA cases, regardless of SNR.
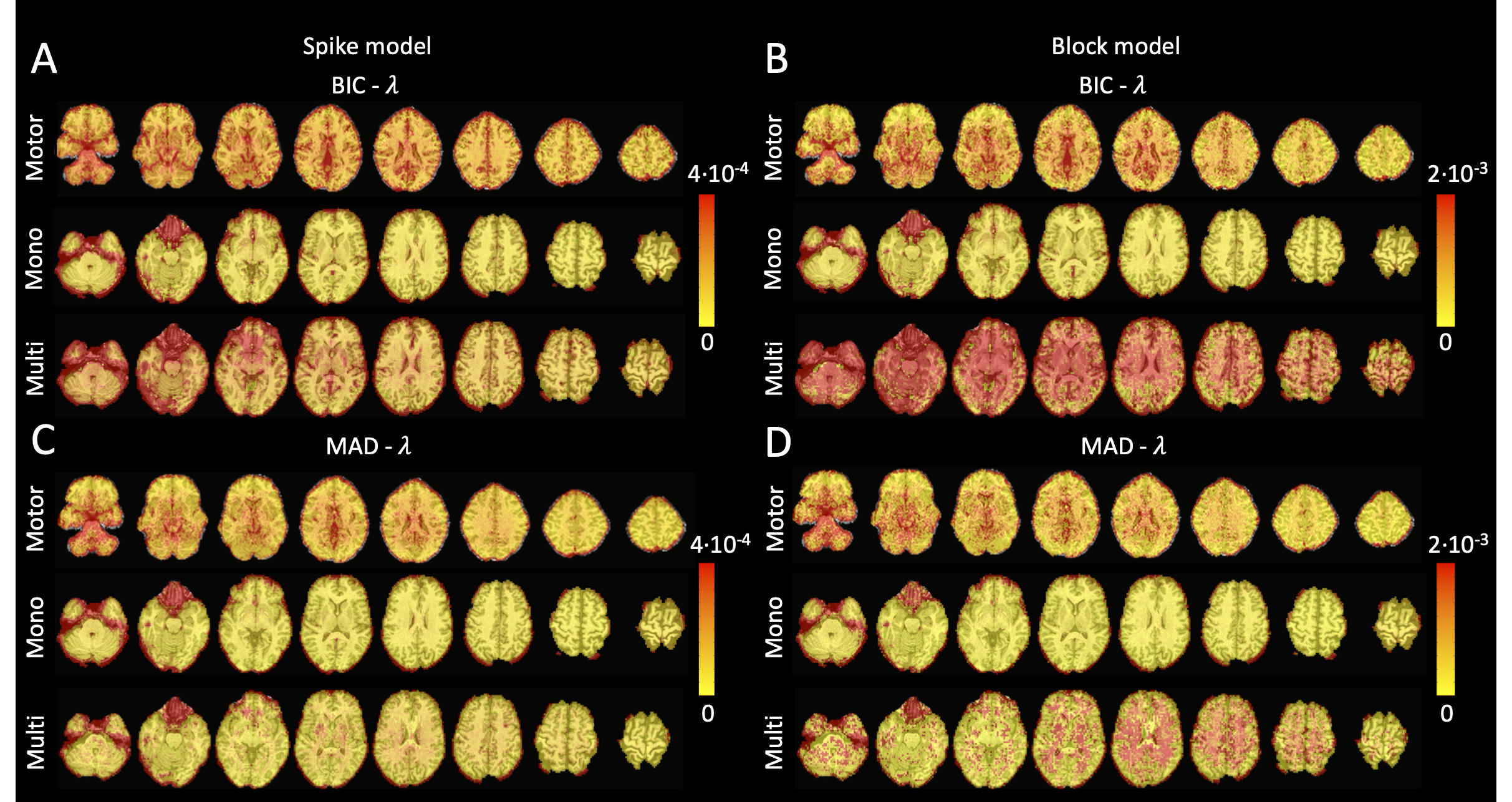
Figure 4:Values of λ across the different voxels in the brain used to estimate (A) the activity-inducing signal (spike model) and (B) the innovation signal (block model) with the BIC selection, as well as (C) the activity-inducing signal (block model) and (D) the innovation signal (block model) with a MAD-based selection. The λ maps are shown for the three experimental fMRI datasets: the motor task (Motor), the monoband resting-state (Mono), and the multiband resting-state (Multi) datasets.
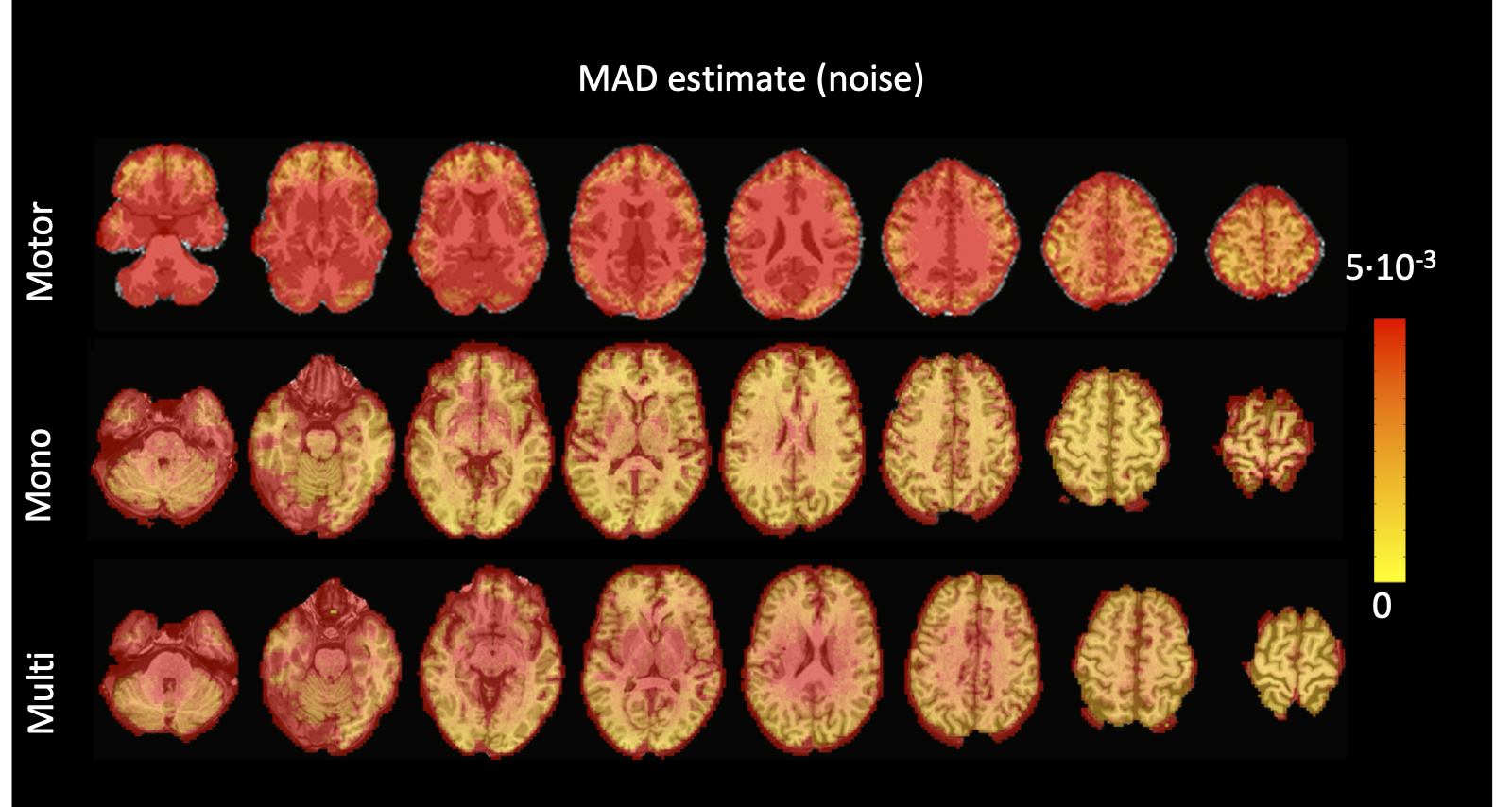
Figure 5:Values of the MAD estimate of standard deviation of the noise across the different voxels in the brain for the three experimental fMRI datasets: the motor task (Motor), the monoband resting-state (Mono), and the multiband resting-state (Multi) datasets.
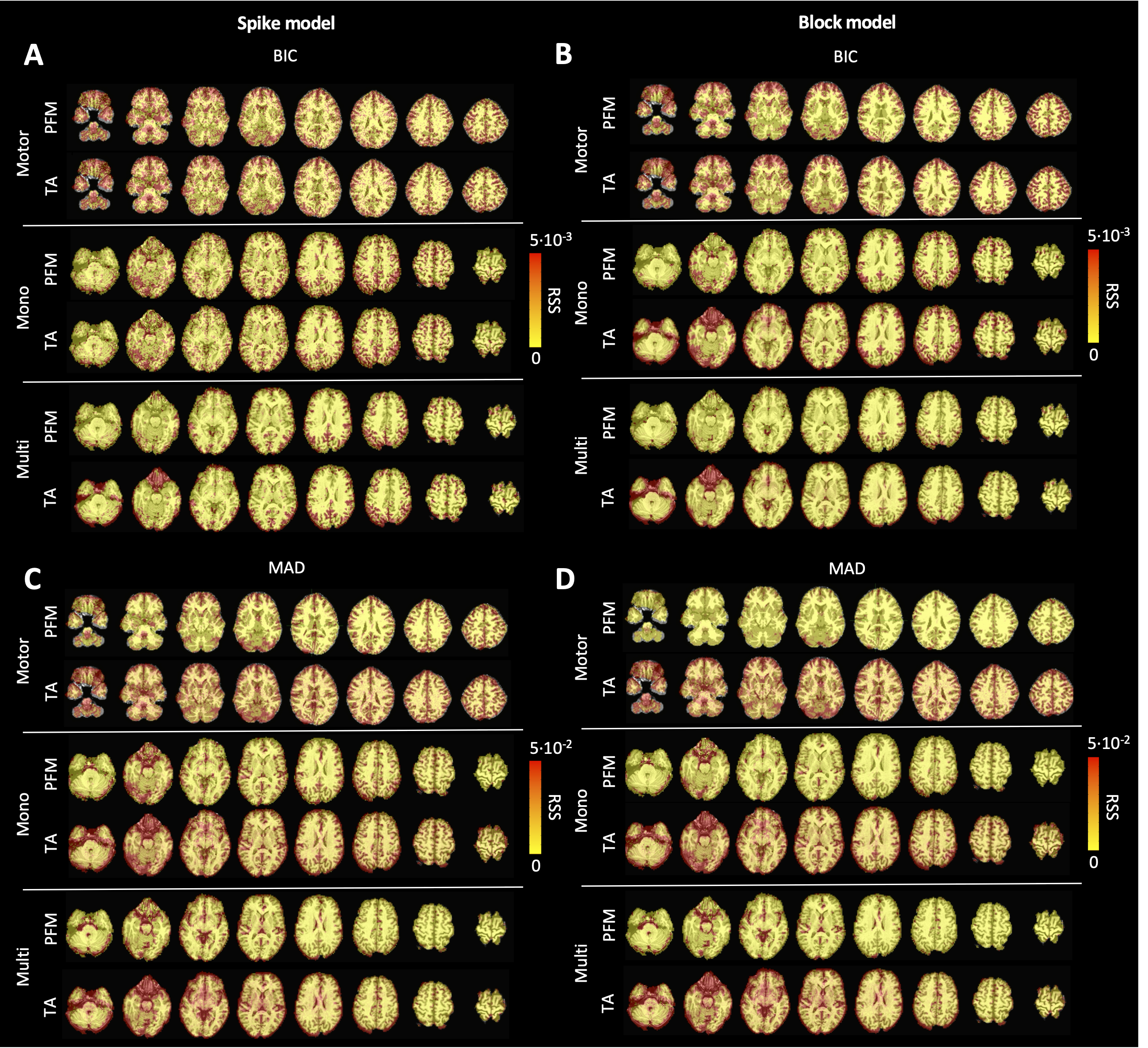
Figure 6:Root sum of squares (RSS) comparison between Paradigm Free Mapping and Total Activation for the three experimental fMRI datasets: the motor task (Motor), the monoband resting-state (Mono), and the multiband resting-state (Multi) datasets. RSS maps are shown for the spike (left) and block (right) models solved with a selection of λ based on the BIC (top) and MAD (bottom) criteria.
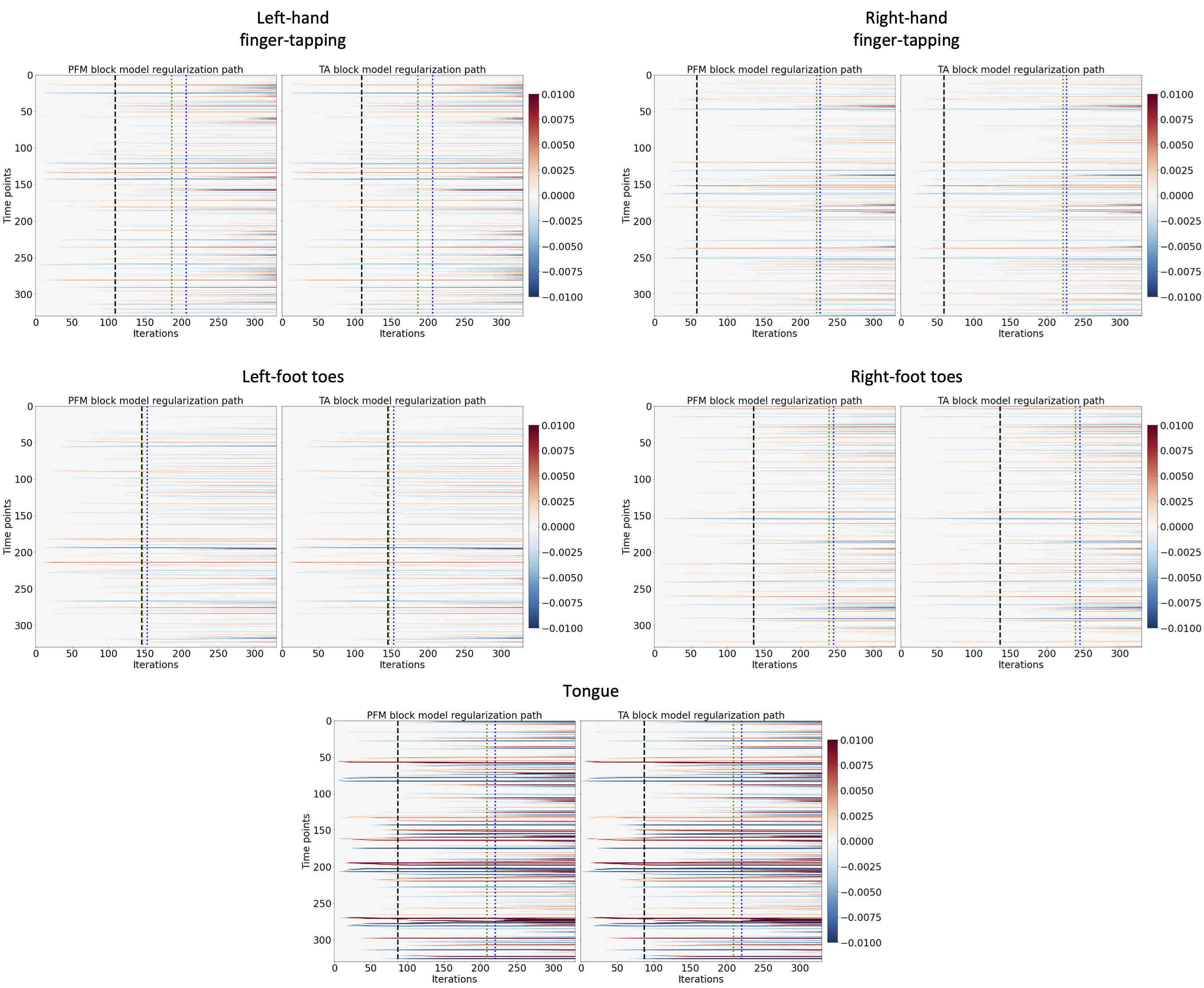
Figure 7:Regularization paths of the innovation signal estimated with PFM and TA as a function of λ (increasing number of iterations in x-axis, whereas each row in the y-axis shows one time-point) for the representative voxels of the motor task shown in Figure \cref{fig:task_maps}. Vertical lines denote selections of λ corresponding to the BIC (black), MAD based on LARS residuals (blue) and MAD based on FISTA residuals (green) optima.
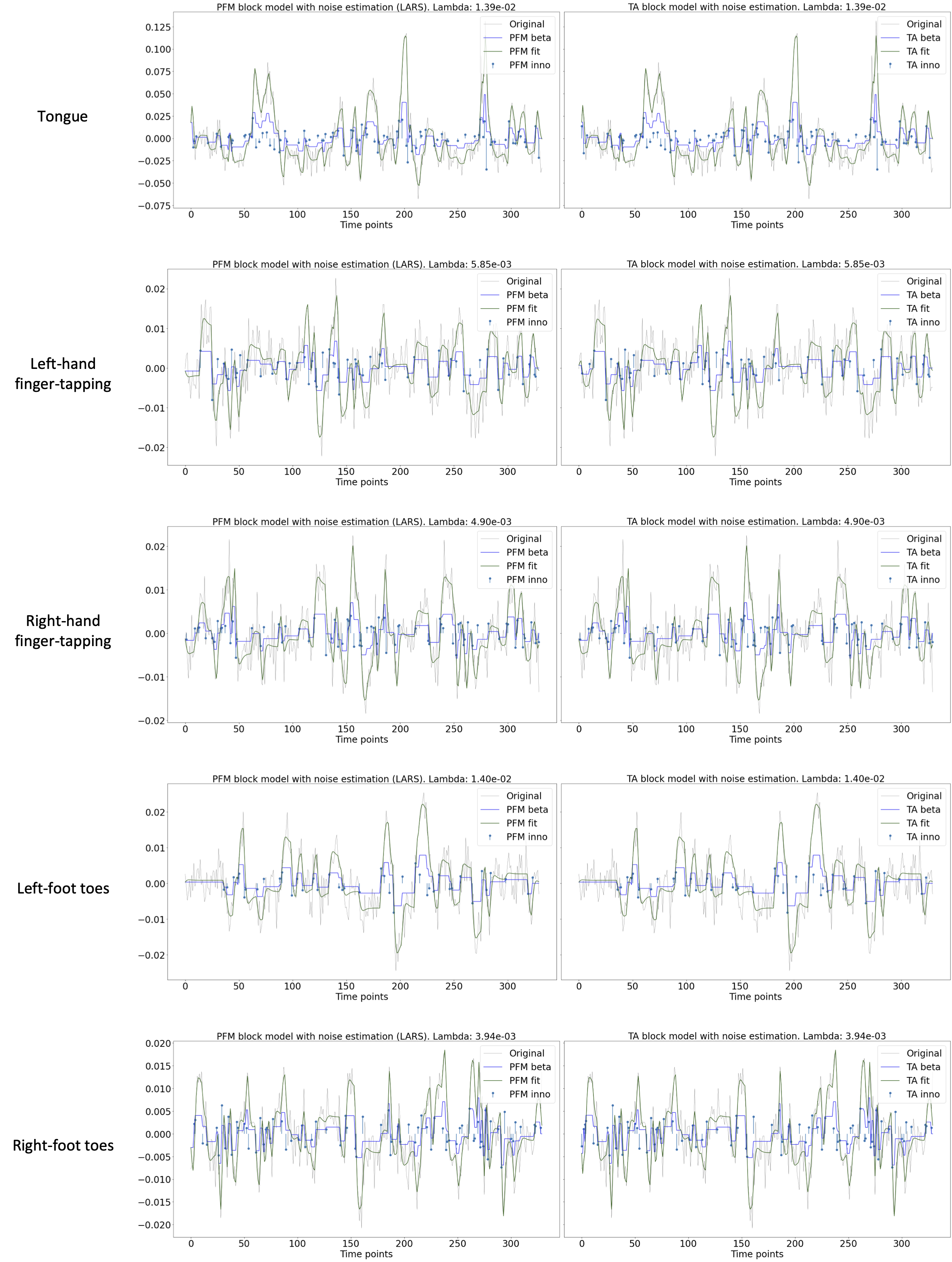
Figure 8:Estimated innovation signal (blue) and activity-related signal (green) for the representative voxels of the motor task shown in Figure~\cref{fig:task_maps} with the MAD selection of λ made by TA, i.e., employing the same λ with both PFM and TA.
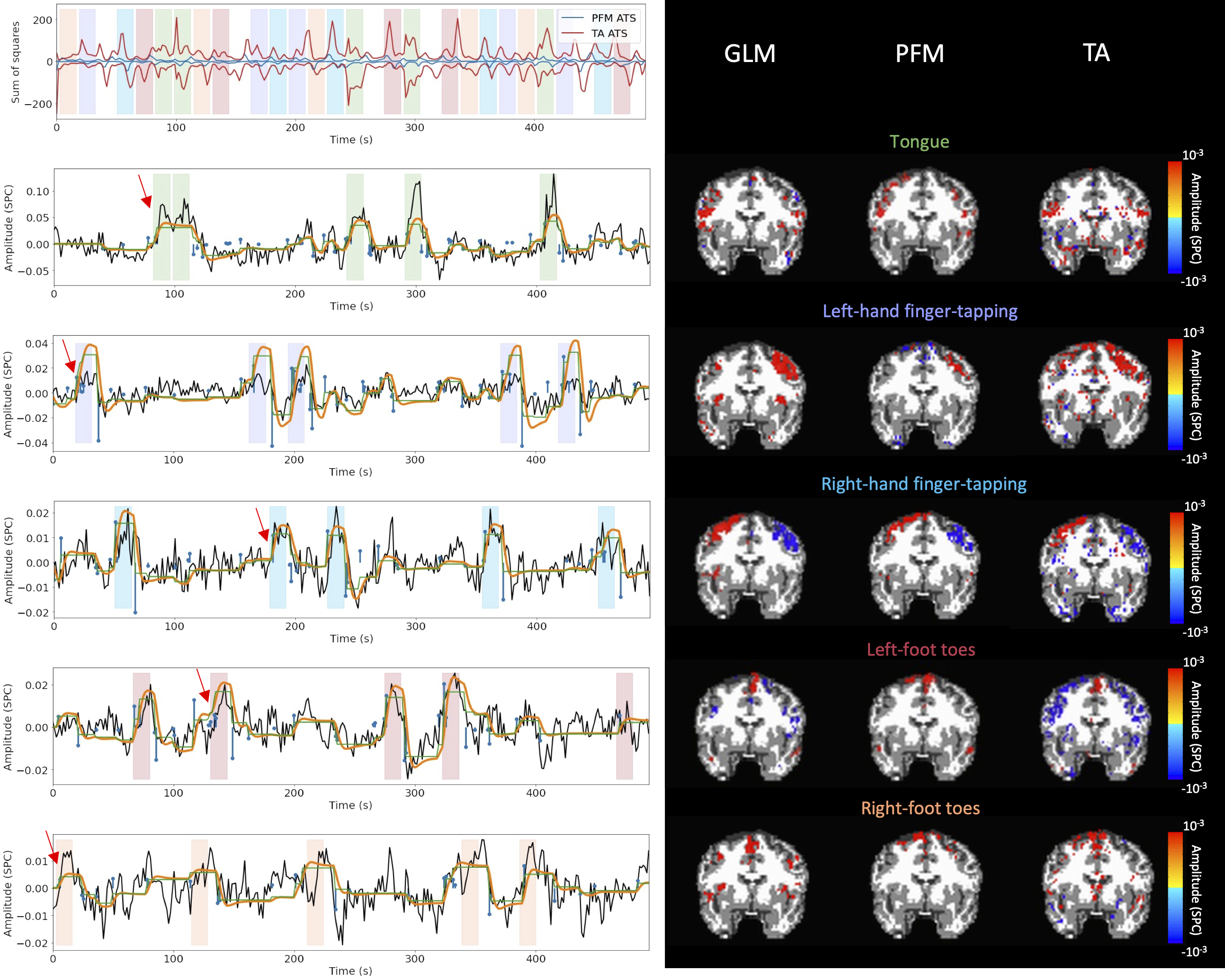
Figure 9:Activity maps of the motor task using a seletion of λ based on the MAD estimate. Row 1: Activation time-series of the innovation signals estimated by PFM (in blue) or TA (in red) calculated as the sum of squares of all voxels at every timepoint. Positive-valued and negative-valued contributions were separated into two distinct time-courses. Color-bands indicate the onset and duration of each condition in the task (green: tongue, purple: left-hand finger-tapping, blue: right-hand finger-tapping, red: left-foot toes, orange: right-foot toes). Rows 2-6: time-series of a representative voxel for each task with the PFM-estimated innovation (blue), PFM-estimated activity-inducing (green), and activity-related (i.e., fitted, orange) signals, with their corresponding GLM, PFM, and TA maps on the right. The maps shown on the right are sampled at the time-point labeled with the red arrows and display the innovation signals at that moment across the whole brain.
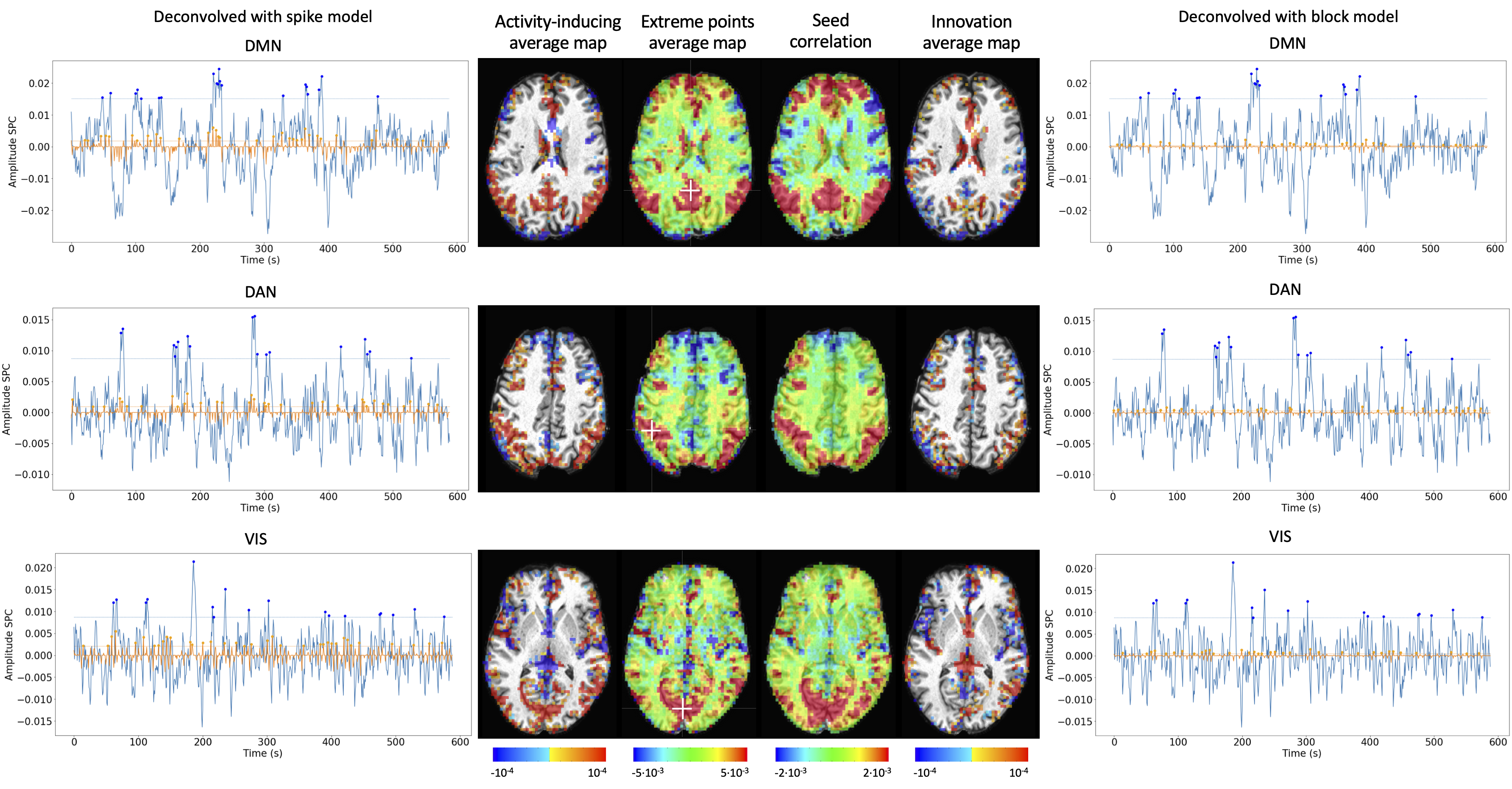
Figure 10:Activity-inducing CAPs (left) and innovation CAPs (right) obtained with the PFM-estimated activity-inducing and innovation signals respectively, using a MAD-based selection of λ. Time-points selected with a 95th percentile threshold are shown over the average time-series (blue) in the seed region (white-cross) and the deconvolved signal (orange). CAPs and seed correlation maps are illustrated in the center.